Advancing Predictive Maintenance: A Machine Learning Approach to Power Converter Reliability
Degradation of power electronics components, such as DC-link capacitors in three-phase inverters, poses significant challenges for maintaining reliability and efficiency in modern power systems. Extending the lifespan of power converters and ensuring their safe and efficient operation comes through the identification and mitigation of this degradation. During this secondment, we focused on parameter identification for aging power converters using a system-level condition monitoring framework integrated with machine learning techniques.
The core objective of this work was to develop a condition monitoring framework capable of estimating the degradation of DC-link capacitors, which are among the most failure-prone components in three-phase inverters. By reducing the number and types of sensors required and minimizing sampling requirements, this approach aims to provide a more cost-effective and scalable solution for real-world applications.
One key challenge was to discern capacitor degradation levels across varying operating conditions, including different temperatures, loads, initial voltages, and current distributions, only from system-level signals that are already acquired for control purposes. Traditional condition monitoring methods can suffer from false positives and negatives, particularly when external factors like lower initial voltages affect acquired sequences the same way a reduced capacitance would. Our framework was designed to address these challenges by being robust to such confounding variables, paving the way for more reliable diagnostics.
Our initial efforts focused on analyzing an experimental dataset, from which we developed a machine learning pipeline capable of accurately distinguishing between healthy and degraded capacitors across a range of operating conditions. This pipeline demonstrated its robustness, with results that have been submitted for publication in a conference paper.
Building on this foundation, we explored the use of high-fidelity simulations to replace costly experimental data. By leveraging synthetic data generated through simulations, we aim to reduce dependency on expensive experimental datasets while maintaining the accuracy of condition monitoring. The simulations also open the door to broader studies of parameter variation and degradation mechanisms, which are otherwise challenging to replicate in physical setups.
Looking ahead, we plan to adapt a developed physics-informed machine learning framework for use with synthetic datasets and tailor it to the operating conditions we have experimentally. This integration of physical insights into the machine learning pipeline is expected to enhance generalization capabilities and provide a deeper understanding of the degradation mechanisms.
This secondment provided valuable opportunities for interdisciplinary collaboration and knowledge exchange, across system-level condition monitoring, machine learning, and high-fidelity simulations. We have laid the groundwork for further collaboration, building upon the progress made during the secondment.
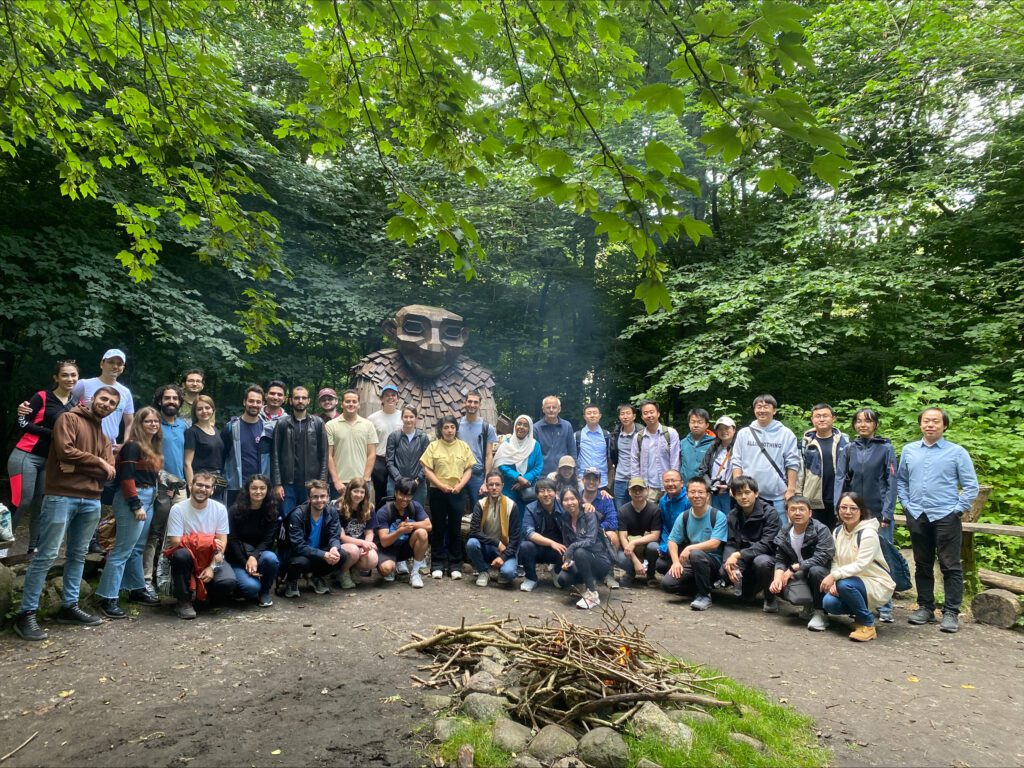