Mobility electrification plays a critical role in the economy decarbonisation, and we are on the edge of a revolution linked to the massive deployment of the electric vehicle (EV). The EV can now replace the internal combustion engine (ICE) vehicle in terms of service provided, supporting the EU’s ambitions for decarbonisation. Besides the reduction of critical material, and decrease of cost, optimising the lifetime of the EV components is essential to accelerate their adoption, especially the powertrain sub-components that have the major impact on EV cost and CO2 emissions. A new-generation of diagnostic and prognostic systems for the powertrain will be a game changer to ensure EV adoption, because they will estimate its degradation, anticipate failures, and ease reparability thus extending its lifespan.
Development of predictive maintenance (PdM) methods for the sub-components of the EV powertrain (battery, fuel cell, e-motor, power electronics) is at the heart of TEAMING, benefitting from recent innovation in sensors, complex modelling techniques and AI-supported data processing methods.
Thanks to international staff exchanges, TEAMING will significantly improve the different facets of the PdM solution: sensors, modelling, Digital Twins, adapted AI, and Physics-Informed Machine Learning methods are at the centre of the studies and present a major potential in term of innovation. TEAMING will advance PdM system to better diagnose the internal physical phenomena of the different EV powertrain components and optimise their performance, lifetime, safety, and reliability.
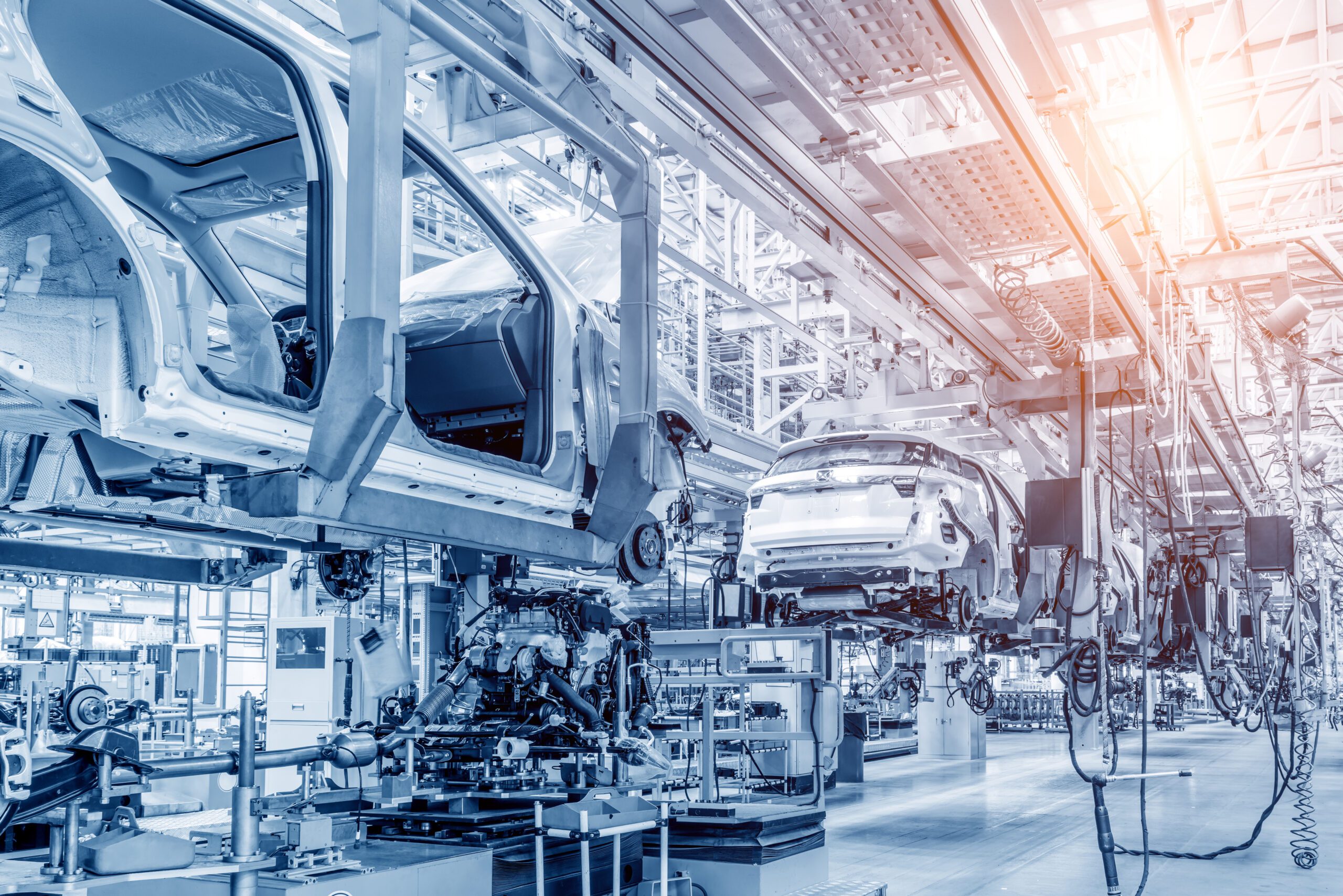
Research Axis 1:
Sensor Improvement
In-operando monitoring of different sub-system states indicators to select a suitable sensor technology.
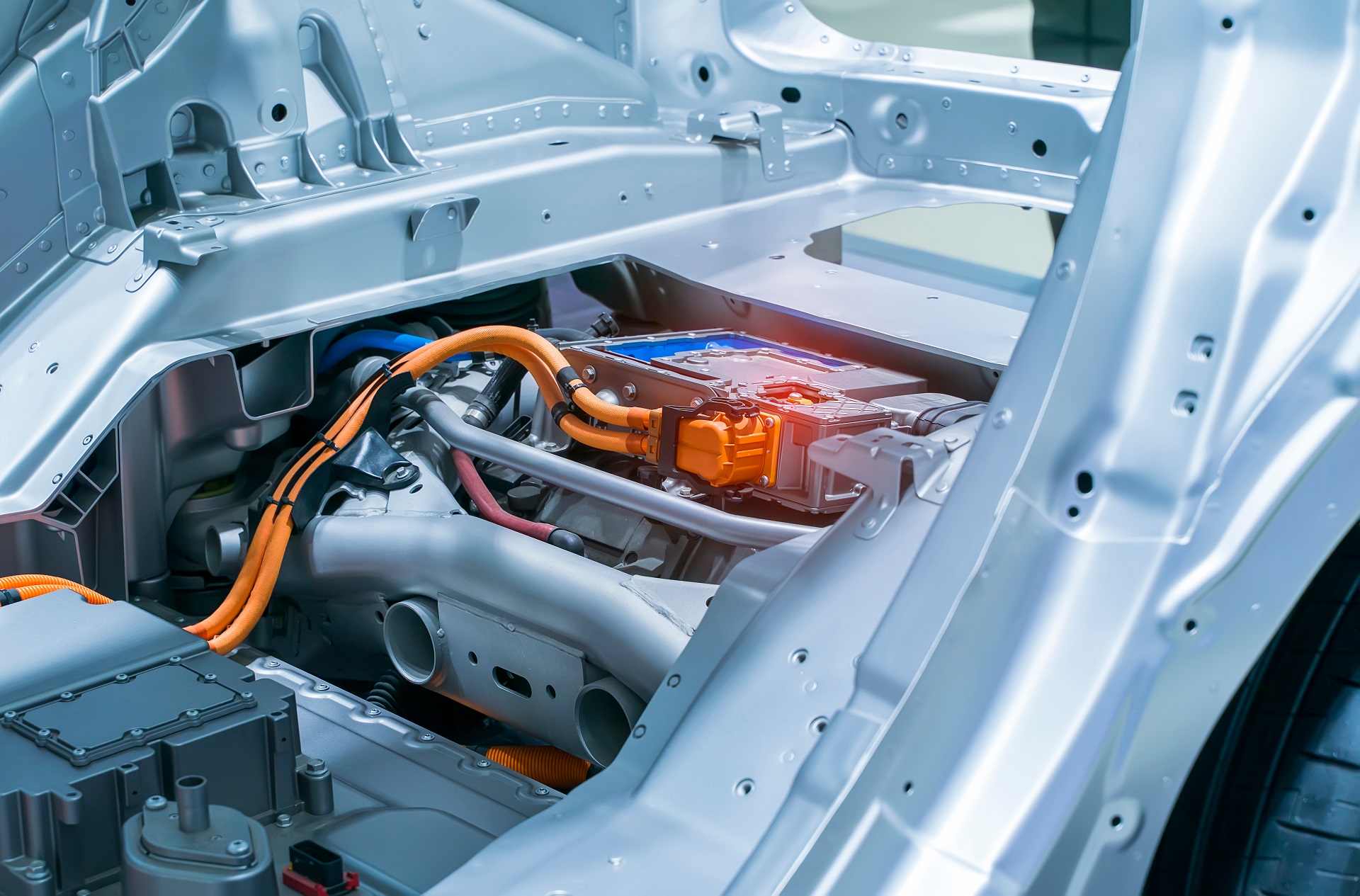
Research Axis 2:
Physics Models Improvement
Design a Digital Twin model representative of the whole powertrain system by accurate modelling of sub-components.
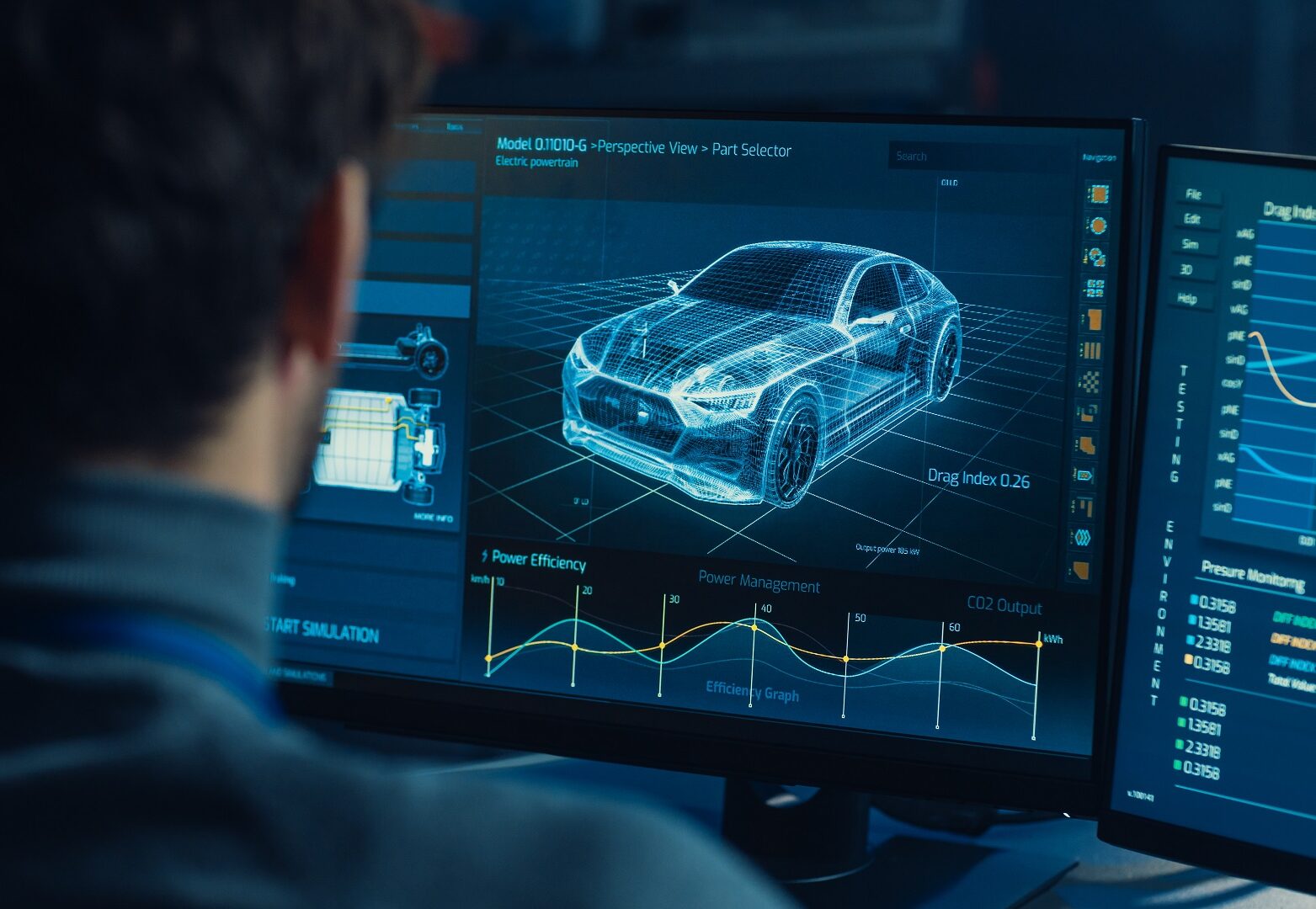
Research Axis 3:
Physics-informed Machine Learning
Lorem ipsum dolor sit amet, at mei dolore tritani repudiandae. In his nemore temporibus consequuntur, vim ad prima vivendum.
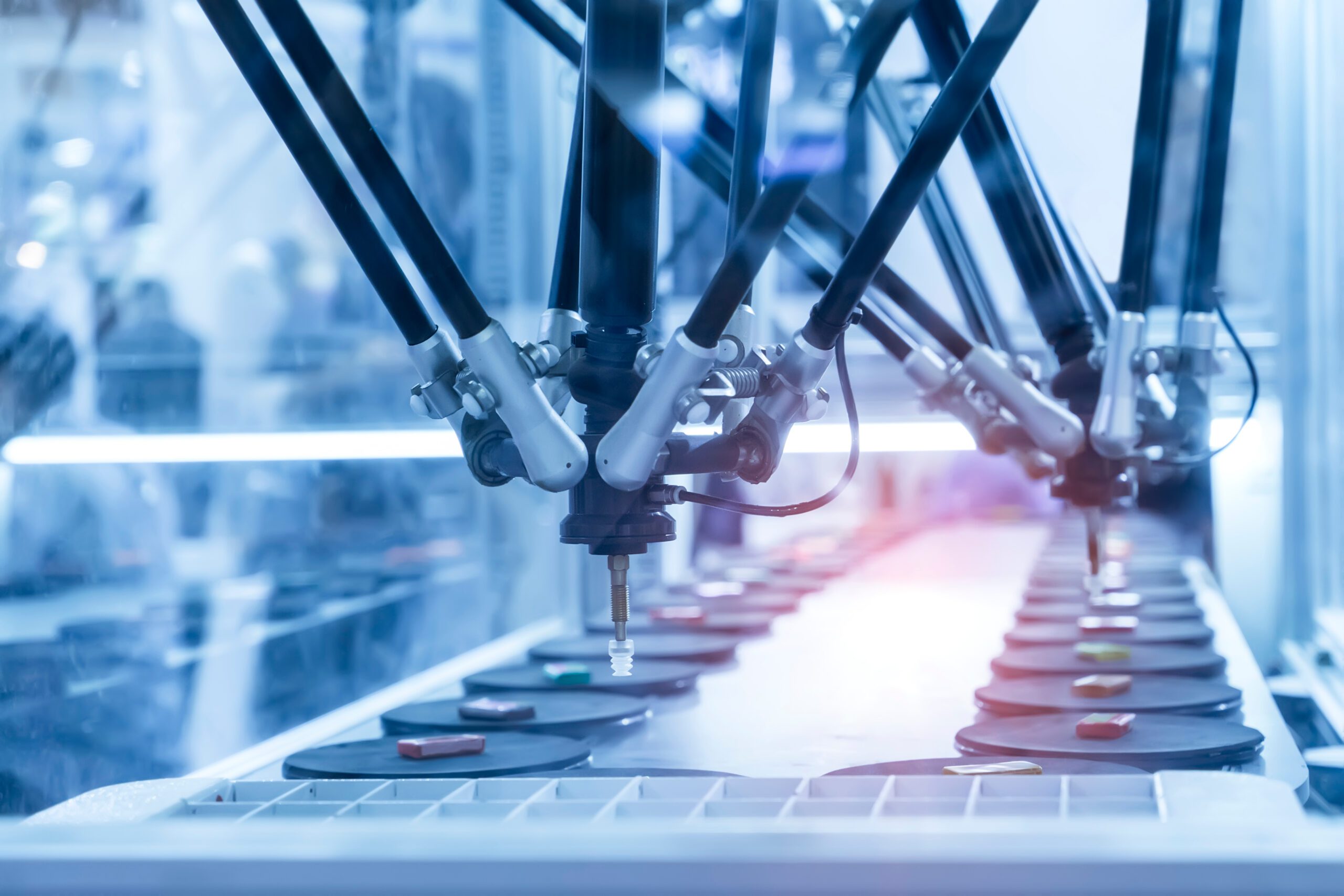
Research Axis 4:
Hybrid methods for predictive maintenance
Targeting embedded systems and digital twins for reliable Remaining Useful Life predictions.
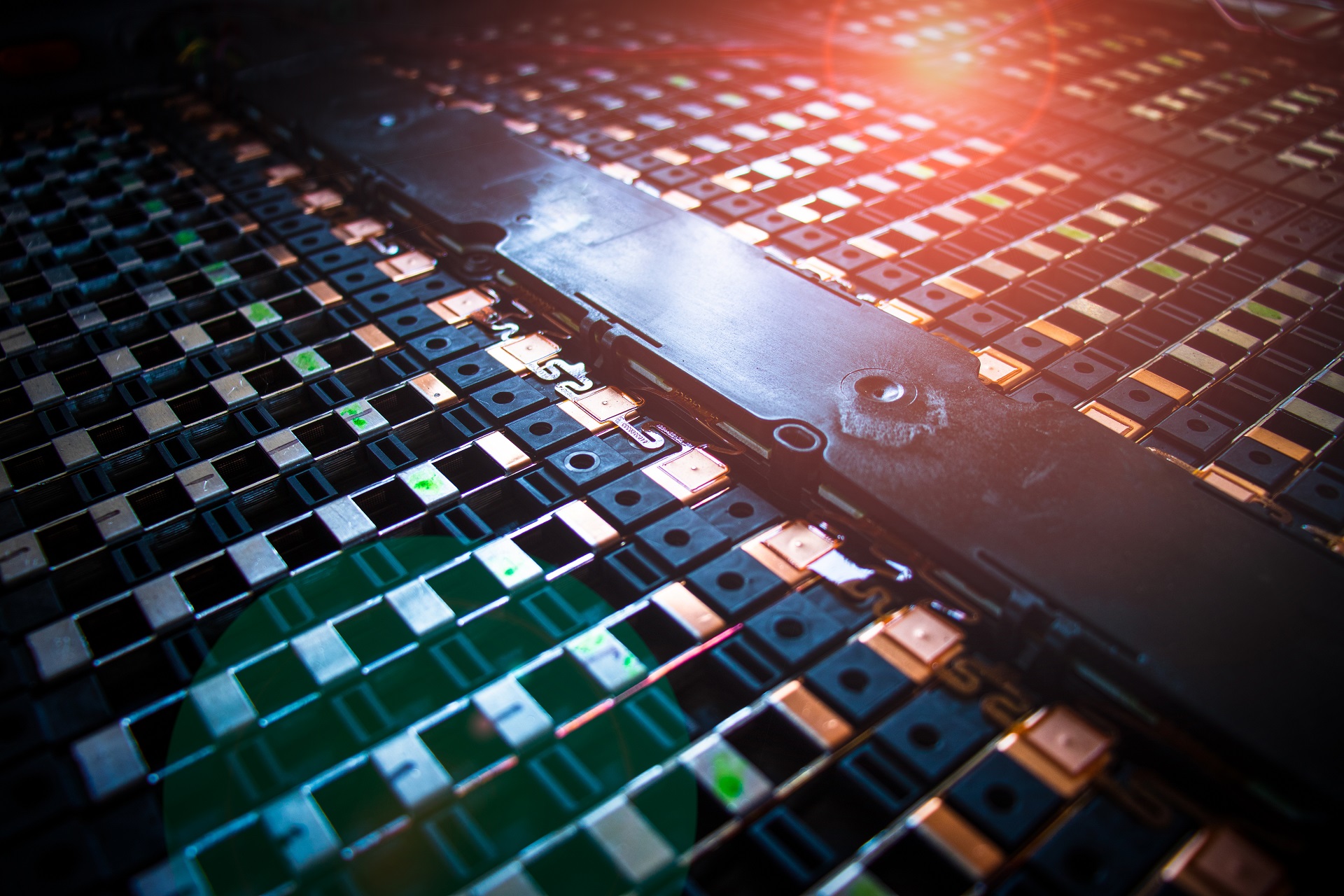
Research Axis 5:
Life Cycle Assessment
Integration of Life Cycle Assessment for optimal usage, eco-design and contribution to circular economy.